Machine Learning in Network Monitoring: A Game Changer for Network Management
Posted on 31 October, 2023 by RUCKUS Networks
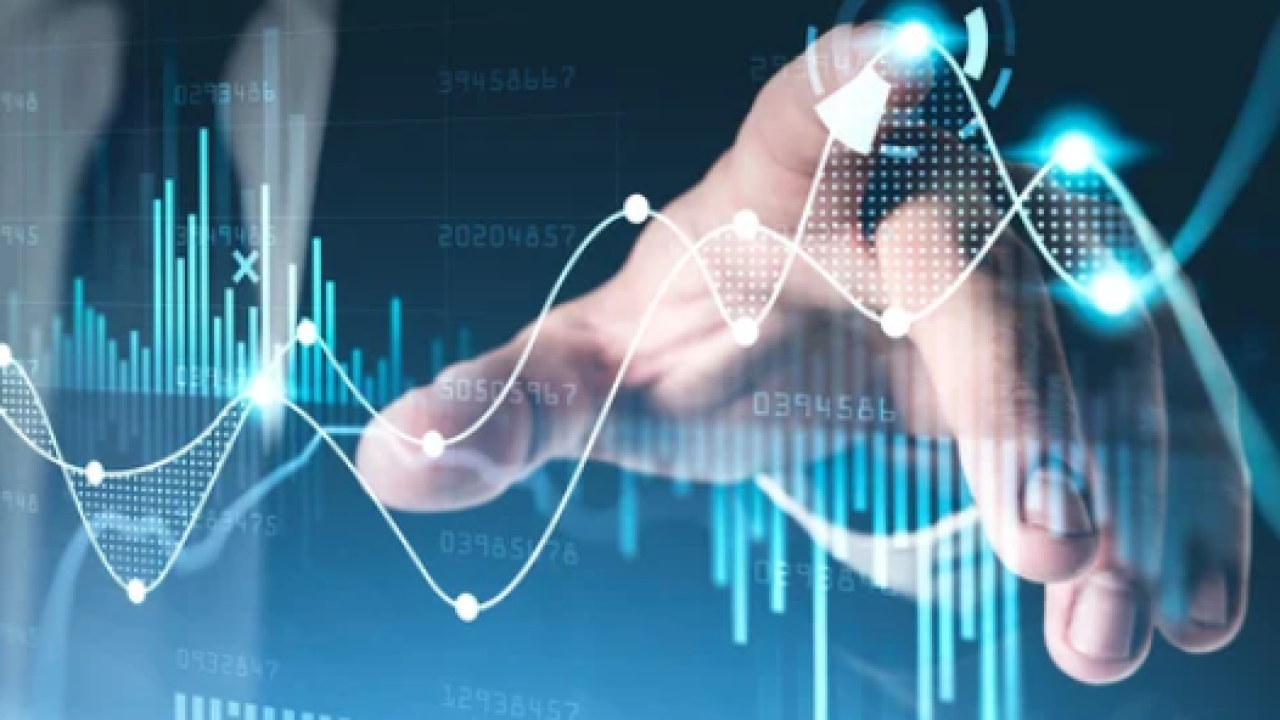
In an era of increasingly complex and dynamic networks, machine learning (ML) has emerged as a powerful tool in the realm of network monitoring and management. ML network monitoring leverages algorithms and predictive analytics to provide real-time insights, proactively identify issues, and enhance network performance. This article delves into the significance and impact of ML network monitoring.
The Role of ML in Network Monitoring:
ML network monitoring goes beyond traditional monitoring methods, offering the following essential features:
1. Anomaly Detection: ML algorithms continuously analyze network data to identify unusual patterns and anomalies that may indicate network issues or security threats.
2. Predictive Analytics: ML models use historical data to predict future network behavior, helping administrators make informed decisions and allocate resources efficiently.
3. Automation: ML-driven systems automate routine tasks like network configuration, reducing the workload on IT teams.
Key Benefits of ML Network Monitoring:
1. Enhanced Security: ML algorithms can identify irregular network behavior, aiding in the early detection of potential cyber threats or vulnerabilities.
2. Proactive Issue Resolution: ML-driven tools can identify network issues before they impact operations, ensuring minimal downtime.
3. Improved Performance: ML models optimize network traffic and resource allocation, enhancing user experience.
4. Advanced Analytics: ML network monitoring provides deep insights into network data, enabling administrators to make data-driven decisions.
Challenges and Considerations:
1. Data Volume: ML requires substantial amounts of high-quality data for training and ongoing monitoring.
2. Complexity: Implementing ML network monitoring solutions may require significant technical expertise and resources.
3. Cost: While ML monitoring can lead to long-term cost savings, the initial investment in technology and training can be substantial.
Use Cases of ML Network Monitoring:
1. Network Traffic Analysis: ML models can analyze traffic patterns to understand how users and devices interact with the network.
2. Predictive Maintenance: ML can predict when network components might fail, enabling preventive maintenance.
3. Quality of Service (QoS): ML can optimize network traffic for different applications to ensure consistent QoS.
4. Resource Allocation: ML-driven systems can allocate network resources dynamically based on current demands.
Conclusion:
Machine learning in network monitoring is reshaping the landscape of network management. Its ability to proactively detect anomalies, enhance security, and optimize performance is invaluable in today's rapidly evolving digital ecosystem. ML network monitoring empowers administrators to maintain network reliability, mitigate security risks, and meet the growing demands of users and applications. As networks continue to evolve, ML monitoring will remain a critical tool for organizations seeking to stay ahead in the ever-changing world of network management.
For more info. visit us:
https://elceclinics.com/
19 September, 2019