Machine Learning vs. Deep Learning
Posted on 12 October, 2023 by seven yevale
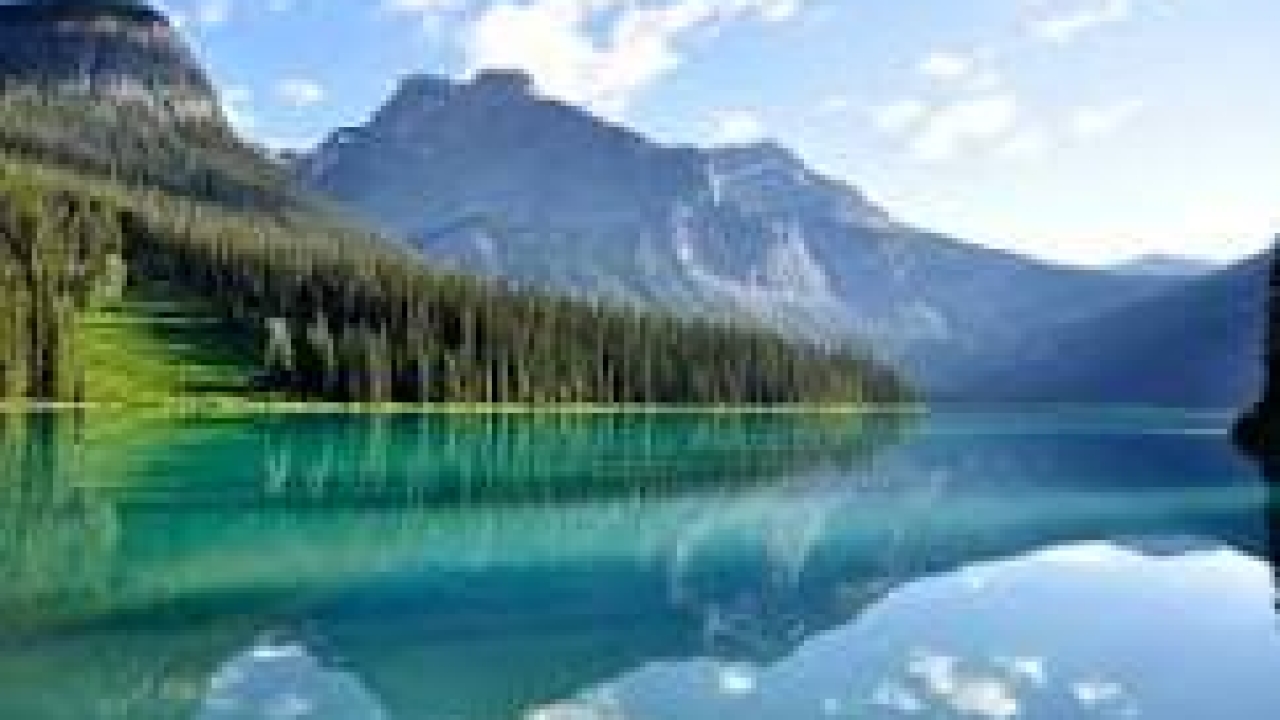
In the realm of artificial intelligence and data science, two terms that often come up are "Machine Learning" and "Deep Learning." These are powerful subfields of AI, but what sets them apart? Why would one choose one over the other for a particular task? In this blog, we'll delve deep into the world of Machine Learning (ML) and Deep Learning (DL), exploring their differences, applications, and the pros and cons of each. Data Science Course in Pune
Machine Learning: The Foundation of Automation
Machine Learning, often considered the older sibling of Deep Learning, is a branch of artificial intelligence that focuses on developing algorithms and models that enable systems to learn and improve from experience. It's the technology behind recommendation systems, fraud detection, and much more. ML can be broadly categorized into three types:
-
Supervised Learning: This is akin to teaching a model with labeled data. It learns patterns and relationships and can predict outcomes based on new, unseen data. Classic examples include image classification and sentiment analysis. Visit Data Science Course in Pune
-
Unsupervised Learning: Here, the model explores data without guidance. It clusters and segments data into patterns, often used in tasks like customer segmentation and anomaly detection.
-
Reinforcement Learning: RL involves an agent making decisions in an environment to maximize a reward. It's widely used in robotics, gaming, and autonomous systems.
Deep Learning: The Power of Neural Networks
Deep Learning, a subset of Machine Learning, focuses on artificial neural networks and is the technology responsible for many recent AI breakthroughs. The term "deep" refers to the use of multiple layers (deep architectures) within neural networks. Here are some key characteristics:
-
Neural Networks: Deep Learning heavily relies on neural networks, which are inspired by the human brain. These networks can have many hidden layers, allowing them to model complex patterns.
-
Feature Learning: Deep Learning is great at feature learning, meaning it can automatically extract relevant features from raw data. This is particularly beneficial in computer vision, natural language processing, and speech recognition.
-
Massive Data: Deep Learning thrives on big datasets. The more data you provide, the better it can learn and generalize. This is one reason why it's ideal for applications like image and speech recognition.
Machine Learning vs. Deep Learning: The Differences
-
Data Dependency: One of the major distinctions is their data dependencies. ML models require engineered features, while DL models can learn useful representations from raw data. For complex tasks where feature engineering is challenging, Deep Learning shines.
-
Computation and Hardware: Deep Learning is computationally intensive, requiring powerful hardware like GPUs or TPUs. Machine Learning models, being less complex, are often more feasible for simpler hardware setups.
-
Interpretability: Machine Learning models are generally more interpretable, making them preferred for tasks where understanding the model's decisions is crucial. Deep Learning models, with their many layers, can be difficult to interpret.
-
Data Size: Deep Learning models often require extensive amounts of data to generalize well, while Machine Learning models can be effective with smaller datasets.
Applications of Machine Learning and Deep Learning
- Machine Learning Applications:
- Recommendation Systems: Think Netflix, Amazon, or Spotify recommendations.
- Credit Scoring: Determining creditworthiness based on historical data.
- Text Classification: Sentiment analysis, spam detection, and content categorization.
- Anomaly Detection: Identifying fraudulent transactions or network intrusions.
- Deep Learning Applications:
- Computer Vision: Object recognition, image segmentation, and facial recognition.
- Natural Language Processing: Language translation, chatbots, and text generation.
- Speech Recognition: Voice assistants like Siri or Google Assistant.
- Autonomous Systems: Self-driving cars, drones, and robotics.
The Pros and Cons
-
Machine Learning Pros:
- Interpretability
- Efficiency with small data
- Suitable for structured data
- Requires less computational power
-
Machine Learning Cons:
- Feature engineering can be time-consuming
- Limited performance on complex tasks
-
Deep Learning Pros:
- Exceptional performance on complex tasks
- Feature learning from raw data
- State-of-the-art results in many domains
-
Deep Learning Cons:
- High computational requirements
- Data-hungry
- Limited interpretability
Conclusion: Choose Wisely
In the realm of Machine Learning vs. Deep Learning, there's no one-size-fits-all answer. The choice between the two depends on the problem at hand. If you have a wealth of data and face complex tasks, Deep Learning may be your best friend. However, if interpretability or efficiency with smaller datasets is crucial, Machine Learning can be the more prudent choice. In many real-world scenarios, a combination of both approaches is used to harness their respective strengths.
As AI and data science continue to evolve, understanding the differences between Machine Learning and Deep Learning is essential for making informed decisions about which technology to leverage for your specific applications.