What is the confusion matrix?
Posted on 23 May, 2023 by Gurpreetsingh
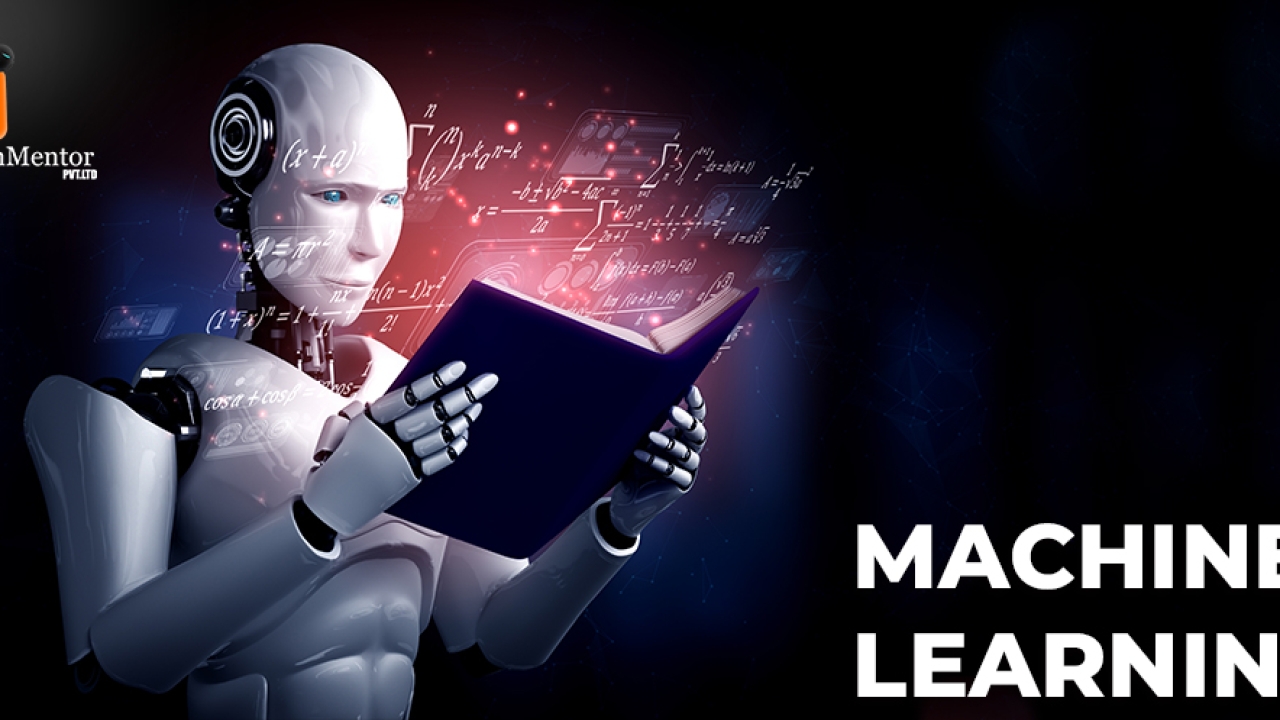
Presentation
Machine learning calculations have become progressively predominant in different fields, including information examination, picture acknowledgment, and regular language handling. One vital part of assessing the exhibition of these calculations is precision. Precision is a major metric that actions the dependability and rightness of forecasts made by a machine learning model. In this article, we will dive into the idea of precision, investigate its computation techniques, and feature its importance in surveying the viability of machine learning models. Machine Learning Course in Pune
I. Characterizing Exactness
Exactness, with regards to machine learning, addresses the proportion of accurately anticipated occurrences to the all out number of examples in a dataset. It gives a sign of how well a model is performing by estimating its capacity to characterize or foresee results accurately. Exactness is ordinarily communicated as a rate going from 0% to 100 percent, where higher qualities demonstrate better execution.
II. Estimation of Exactness
To ascertain exactness, one should contrast the model's expectations and the genuine known values. The cycle includes separating the quantity of accurately anticipated occurrences (genuine up-sides and genuine negatives) by the complete number of examples in the dataset. The equation for exactness is as per the following:
Precision = (TP + TN)/(TP + TN + FP + FN),
where:
TP (Genuine Up-sides) alludes to the quantity of occurrences accurately anticipated as sure.
TN (Genuine Negatives) addresses the quantity of cases accurately anticipated as negative.
FP (Misleading Up-sides) is the quantity of occurrences anticipated as certain however are really negative.
FN (Bogus Negatives) signifies the quantity of occurrences anticipated as negative however are really sure.
III. Meaning of Precision
Precision is a crucial measurement in machine learning as it gives a far reaching outline of the model's general presentation. Its significance lies in a few viewpoints:
Execution Assessment: Precision assists with evaluating how well a model acts in making right forecasts. By taking into account the extent of exact outcomes, engineers and analysts can look at changed models or varieties of a similar model to decide the best one. Machine Learning Classes in Pune
Model Determination: Exactness is in many cases used to analyze and choose the best model among various other options. It permits leaders to pick the model that yields the most noteworthy exactness rate, guaranteeing the most dependable expectations.
Benchmarking: Exactness goes about as a benchmark to assess the advancement and improvement of machine learning models over the long run. By contrasting the exactness of more up to date models with past ones, scientists can gauge headways and recognize regions for additional improvement.
Imbalanced Datasets: In situations where datasets have imbalanced class disseminations, precision alone probably won't give a complete assessment. In such cases, extra measurements like precision, recall, or F1 score can be utilized to acquire further experiences into model execution.
Space Explicit Contemplations: Precision's importance might change relying upon the application area. For example, in clinical conclusion, accurately distinguishing positive cases (responsiveness) may be more basic than generally precision. Understanding space explicit prerequisites is significant when deciphering exactness in setting. Machine Learning Training in Pune
End
Exactness fills in as a crucial measurement for assessing the exhibition of machine learning models. By working out the proportion of right forecasts to the all out number of examples, exactness offers bits of knowledge into the unwavering quality and adequacy of models across different areas. Nonetheless, it is essential to take note of that precision alone may not necessarily give a total picture, especially in cases including imbalanced datasets or explicit space necessities. Subsequently, specialists ought to consider extra assessment measurements close by exactness to guarantee thorough examination and informed dynamic in machine learning applications.
http://www.batterybhai.com
4 April, 2019